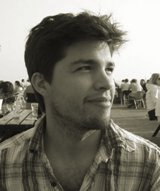
About me
I work as Lead AI/ML Scientist at OraQ AI where I apply AI and ML to improve patient health in the dental industry.
I also work as an Adjunct Assistant Professor at the Canadian Centre for Behavioral Neuroscience in the University of Lethbridge in Canada where I do research in the intersection of Artificial Intelligence, Machine Learning and Neuroscience.
Areas of interest
With the current development of technology and techniques for recording large neuronal populations simultaneously with great spatial and temporal resolution at multiple scales, we are entering an exciting stage in neuroscience where new analysis tools need to be developed in order to make sense of the amount and richness of these data. At the same time, a great deal of development in machine learning has been taking place which can not only provide innovative ways to extract information from neuroscience data, but also, it has potential to provide new insights about how the brain works. I am interested in the understanding of brain functions and mechanisms that can inform the development of new algorithms to process information and in the development and application of new computational tools for the understanding of brain activity.
- Learning and memory
- Artificial Neural Networks
- Artificial Intelligence and Neuroscience
Projects
Experience dependent changes in brain activity at multiple scales
Changes in neuronal activity can be used by the brain to store information about the outside world. In this project we study experience dependent changes in the spatiotemporal dynamics of stimulus evoked cortical responses using optical wide-field imaging and single-unit recordings.
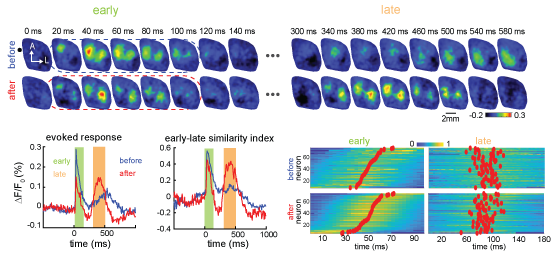
In this work we study how patterns of evoked activity are modified by experience at meso and microcircuit scales using voltage and extracellular glutamate transient recordings over widespread regions of mice dorsal neocortex or single-unit activity recordings with multi-shank silicon probes in rat cortex.
Study of brain activity at multiple scales
The brain is formed of complex networks that communicate at different spatiotemporal scales. In order to study such communication processes, one has to look into brain activity at multiple scales simultaenously.
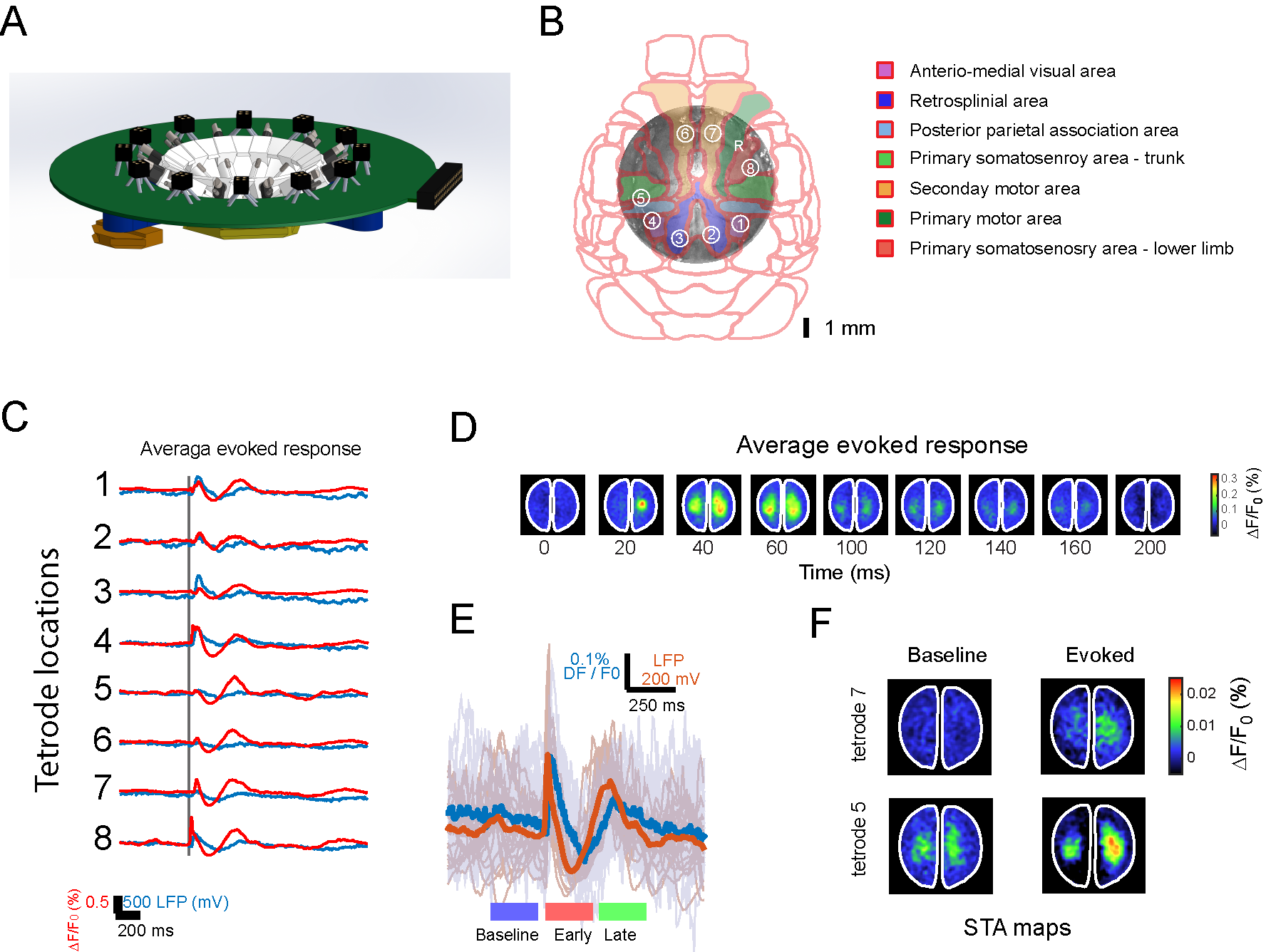
In this paper we recorded brain activity over large cortical areas using VSD wide-field imaging and single-unit activity at multiple brain areas simultaneously.
Reverberation of evoked cortical activity during anaesthesia
Memory and learning are some of the fundamental cognitive processes in animals. Despite continuous research progress in this area, our understanding of memory formation and consolidation processes is far from complete. The study of the neuronal activity implicated in such processes, in many cases, involves long recordings during sleep and awake periods, which in turn, can be complicated because of technological or experimental problems. In this project we show that the hallmarks of memory formation and consolidation can be studied during anaesthesia and that these processes depend on cortical state.
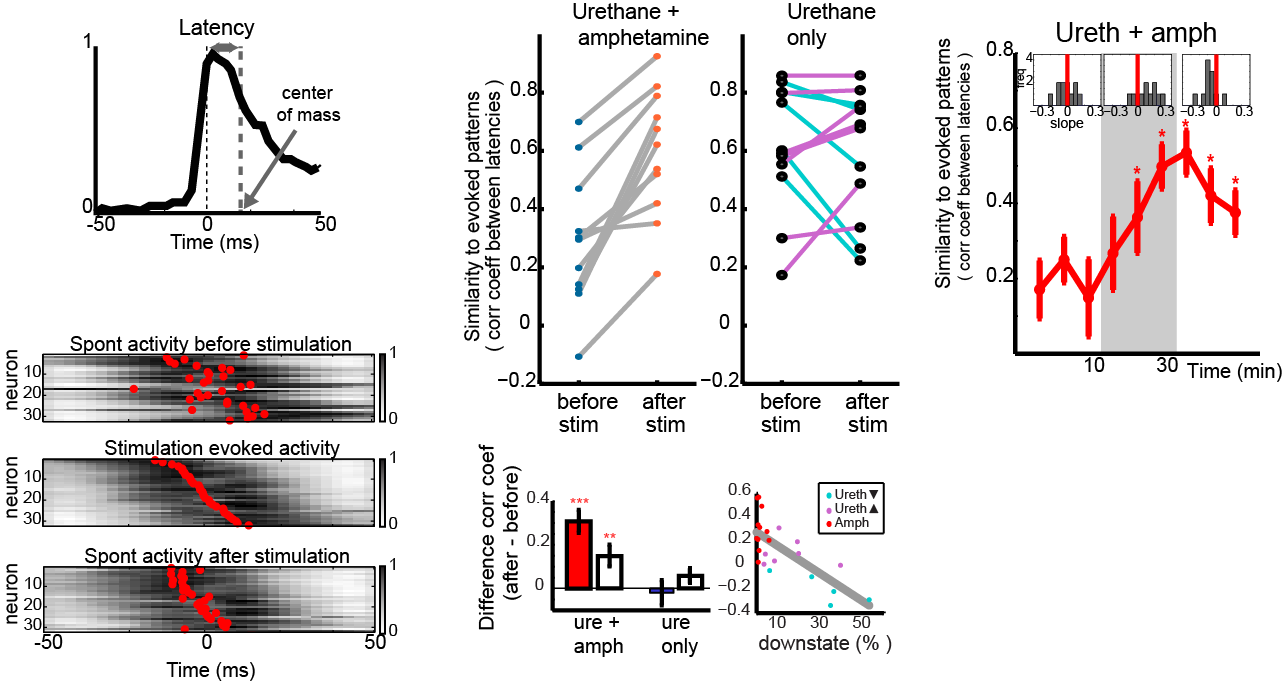
Simulated spatial navigation
Using sensory immediate (egocentric) information from its surroundings, an agent can make predictions about its location in space (allocentric). These predictions can improve the performance of a simulated agent that learns to navigate in a virtual world when similar sensory information is found in the future.
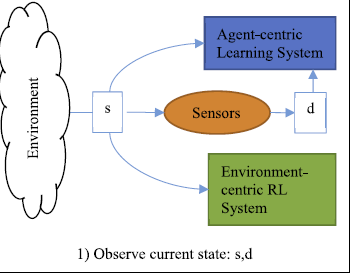
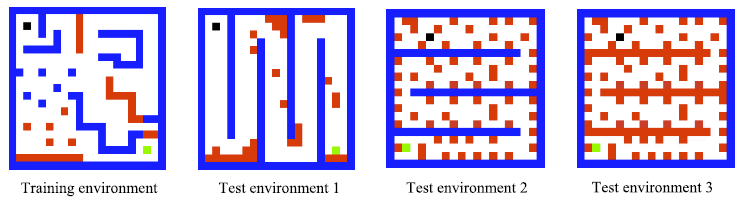
This project uses reinforcement learning to solve a spatial navigation task in a simulated world where an agent exploits sensory-based predictions about its spatial location. A similar strategy can be used not only in spatial tasks, but also in situations where local information can be used to predict consequenses in a more global domain. [paper]
Automated homecage behaviour monitoring
I am interested in developing tools to monitor behaviour and brain activity in the homecage in an automated manner. With the increase in computational power and reduction in costs, microcomputers such as the Raspberry Pi open a great opportunity to design high-throughput systems to study rodent
behavior.
We are currently working to develop such systems and combine them with state-of-the-art machine learning algorithms to learn more about the brain.[paper]
- Bermudez-Contreras, E., Gomez-palacio Schjetnan, A., Luczak, A., and Mohajerani, M. H. Sensory experience selectively reorganizes the late component of evoked responses. Cerebral Cortex(2022)
- Bermudez-Contreras, E., Sutherland, R.J., Mohajerani, M.H., Whishaw, I.Q. Challenges of a small world analysis for the continuous monitoring of behavior in mice. Neuroscience & Biobehavioral Reviews, 2022.
- Navvab Afrashteh, Samsoon Inayat, Edgar Bermudez Contreras, Artur Luczak, Bruce L McNaughton, Majid H Mohajerani. Spatiotemporal structure of sensory-evoked and spontaneous activity revealed by mesoscale imaging in anesthetized and awake mice. Cell Reports, 2021.
- Bermudez-Contreras, E. Deep reinforcement learning to study spatial navigation, learning and memory in artificial and biological agents. Biological Cybernetics, 2021.
- Bermudez Contreras, E., Clark, B.J., Wilber, A. The Neuroscience of Spatial Navigation and the Relationship to Artificial Intelligence. Frontiers in Computational Neuroscience, 2020
- J. Karimi Abadchi, M. Nazari-Ahangarkolaee, S. Gattas, E. Bermudez-Contreras, B.L. McNaughton, M. H. Mohajerani. Spatiotemporal patterns of neocortical activity around hippocampal sharp-wave ripples. eLife, 2020 .
- Ryait H, Bermudez-Contreras E, Harvey M, Faraji J, Mirza Agha B, Gomez-Palacio Schjetnan A, Gruber A, Doan J, Mohajerani M, Metz G.A.S, Whishaw IQ, Luczak A. Data-driven analyses of motor impairments in animal models and neurological disorders. PLoS Biology, 2019.
- Singh, S., Bermudez-Contreras, E., Nazari, M., Sutherland,R.J., Mohajerani, M.H. Low-Cost Solution for Rodent Home-Cage Behaviour Monitoring. PLoS ONE. August, 2019.
- Bermudez-Contreras E, Chekhov S, Tarnowsky J, Sun J, McNaughton BL., Mohajerani MH. High Performance, Inexpensive Setup for Simultaneous Multisite Recording of Electrophysiological Signals and Mesoscale Voltage Imaging in the Mouse Cortex. Neurophotonics 5(2) 2018.
- Chalmers, E., Bermudez Contreras, E., Robertson, B., Luczak, A. Gruber, A. Learning to Predict Consequences as a Method of Knowledge Transfer. IEEE Transactions on Neural Networks and Learning Systems , May, 2017.
- E. Chalmers, E. Bermudez-Contreras, B. Robertson, A. Luczak and A. Gruber, Context-switching and adaptation: Brain-inspired mechanisms for handling environmental changes 2016 International Joint Conference on Neural Networks (IJCNN), Vancouver, BC, 2016, pp. 3522-3529
- E. J. Bermudez Contreras, A. Gomez-Palacio Schjetnan, M. Arif, P. Bartho, B. Kolb, B. L. McNaughton, A. Gruber, A. Luczak. Formation and reverberation of sequential neural activity patterns evoked by sensory stimulation is enhanced during cortical desynchronization. Neuron, Vol. 79, No. 3. (7 August 2013), pp. 555-566.
- Bermudez, E., Buxton, H. and Spier E. Attention can improve a simple model for visual object recognition. Image Vision Comput., 26(6):776.787, 2008.
- Bermudez-Contreras, E. Philippides, A. and Seth, A.K. Movement Strategies for Learning in Visual Recognition. In S. Bullock, J. Noble, R. A. Watson, and M. A. Bedau(Eds.) Proceedings of the 11th International Conference on Artificial Life, Alife XI 2008, Winchester, UK, MIT Press, Cambridge, MA.
- Bermudez-Contreras, E. and Seth, A.K. Simulations of simulations in evolutionary robotics. In Almeida e Costa, F. et al. Proc. 9th European Conference on Artificial Life (ECAL2007), pp.796-806.
- Bermudez, E. A Biologically Inspired Solution for an Evolved Simulated Agent. Proc. Genetic and Evolutionary Computation Conference (GECCO 2007). London, England. July, 2007.